Do L&D pros dream of abundant choice?
How much content do you really need in your learning library?
In Do Androids Dream of Electric Sheep?, the Philip K Dick novel that inspired Blade Runner, J.R. Isidore lives alone in a building awash with decay. He refers to this mess as ‘kipple’:
‘Kipple is useless objects, like junk mail or match folders after you use the last match… When nobody's around, kipple reproduces itself. For instance, if you go to bed leaving any kipple around your apartment, when you wake up the next morning there's twice as much of it.’
Does that sound like your LMS or LXP?
Over the last few years, learning content libraries have grown and grown. Rapid authoring tools made it easy for anyone to make e-learning. The rush to create training for every workplace issue led to more. The cyber security package your IT team bought five years ago sits alongside the ‘Company Values’ module you commissioned in 2016.
When you wake up the next mornining there’s twice as much of it.
At the same time, your people face a variety of workplace challenges. You can’t just have one perfect piece of e-learning that will make them ‘perform better’.
And so the optimal volume of learning content sits somewhere between ‘1’ and ‘kipple’.
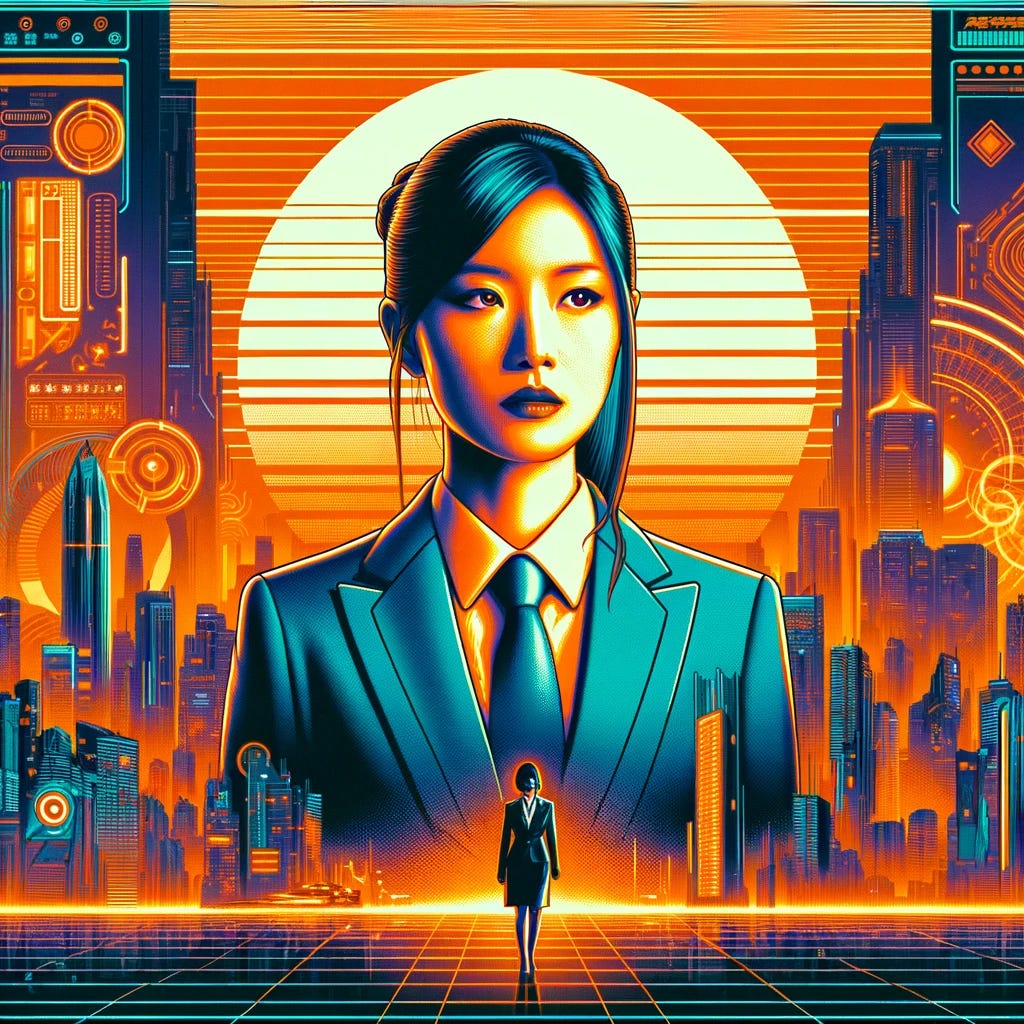
You might be thinking: This isn’t a big deal. Content discovery mechanisms like navigation hierarchies, search bars, content tagging and AI tools make it easy for my people to find the content they need.
But there’s three reasons I think it’s worth a regular clean-up of your content library:
First, you might be paying too much for content that no one uses.
Second, your people may be wading through a sea of kipple in order to find the thing they need.
Third, if your people find out-of-date or low quality content, and think this is representative of your offering, then the chances of them coming back to you are slim.
And then there’s the issue of choice.
Back in 2000, researchers Sheena Iyengar and Mark Lepper ran the now famous ‘Jam Experiment’. On two consecutive weekends, they set up a booth in an upscale grocery store and presented customers with a choice of jam. On one occasion they presented six varieties. On the other, they presented 24.
The results were dramatic. Although the larger selection attracted more customers, the smaller selection led to ten times as many purchases.
It seemed that, when people are presented with too much choice, they’re inclined to give up and walk away.
Then things got sticky.
The Financial Times’ Tim Harford pointed out that this finding doesn’t explain how Starbucks became ubiquitious with 80,000+ drinks combinations. Attempts to replicate the original experiment have failed.
And so it seems that too much choice is bad, but not all the time.
So how to determine the optimum size for your learning content library?
In 2015, a meta-analysis of studies looking at ‘choice overload’ identified four factors that I think are useful for making this determination. According to the researchers, presenting people with fewer options is effective when:
the decision is difficult (there are lots of factors to consider)
the decision is complicated (there is no clear ‘best’ option)
people don’t have any particular preference (they don’t really know what they are looking for)
people have a clear goal (they want a particular outcome and are not just ‘browsing options’)
The short version of this paper is that, the more people have to think about a decision, the less likely they are to make a selection.
This somewhat answers Harford’s point on Starbucks. Yes, there are 80,000+ drinks combinations. But for many customers the decision is easy, there is a ‘best’ option, they have a clear preference and they intend to leave with a coffee. As evidence of this, data from the Starbucks app shows that 65% of customers order the same type of drink each time, and 90% order the same size.
Helping your people overcome workplace challenges or problems is different to asking them their choice of coffee. If they are struggling enough that they’ve turned to your learning library for help, they likely need more guidance to make a decision.
As an example, imagine a new manager is recruiting for the first time. They want to know how to ask good interview questions.
Giving them 20 learning resources on how to do this is counter-productive. It would be better to give them two or three, each with clearly articulated benefits.
So let’s clean up our content libraries.
Our friends at aNewSpring, Roy de Vries and Ger Driesen, advocate doing this once a year on March 21, World Learning Content Clean-Up Day (WLCCD). And research from Mind Tools shows that 87% of top-performing L&D teams remove content that’s no longer relevant, compared to 17% of the lowest-performing teams.
Will you join the cause?
Fight the kipple, optimize your content library, celebrate with jam.
At Mind Tools, we put a real emphasis on managing our content library to make sure that every resource is useful, relevant and targeted at a particular workplace problem. Email custom@mindtools.com or reply to this newsletter from your inbox if you want to find out more.
🎧 On the podcast
Historically, if you wanted to give your managers an opportunity to practice having difficult conversations, you had to facilitate embarrassing roleplays or hire actors. They could only practice once or twice, and it might be weeks or months before a difficult situation comes up when they actually have to use this skill.
As Learning Pool’s Lindsey Coode argues on this week’s episode of The Mind Tools L&D Podcast:
‘Unless they actually get to practice, the first time they're really having this type of conversation is in the real world when there's a real problem within their team. So that's a really high-risk situation.’
Lindsey joined us this week because Mind Tools and Learning Pool have just launched our first collaborative product: AI Conversations.
AI Conversations leverages the power of generative AI to simulate difficult conversations with a variety of characters: some nice, some more prickly.
You can make it available on demand to your people, or as part of a blended learning program.
Listen to the full episode below. 👇
You can subscribe to the podcast on iTunes, Spotify or the podcast page of our website. Want to share your thoughts? Get in touch @RossDickieMT, @RossGarnerMT or #MindToolsPodcast
📖 Deep dive
Speaking of AI, last week Donald H Taylor and Egle Vinauskaite published their report ‘AI in L&D: The State of Play’, the results of an anonymous survey of 185 self-selected respondents recruited via social media.
Full disclosure, I took part in the research and am quoted in the report, but I think there’s a raft of insights here about how L&D teams are actually using AI in practice.
A key finding is that, for now, the most common use cases are in content creation and improving efficiencies. The authors argue that this is to be expected, since tools like ChatGPT make it so easy to generate written copy and images, or to get quick answers to questions.
More sophisticated use cases like personalisation, providing skills practice and maintaining skills taxonomies are lower down the list, but are already being deployed by some teams (see the AI Conversations podcast above, as an example).
If you’re not already using AI in your L&D team, though, take heart. According to the report authors:
‘If there is one message L&D practitioners should take away from this report, it is that there is no need to panic - you are not falling far behind your peers, for the simple reason that very few are making major strides with AI.’
This aligns nicely with a previous L&D Dispatch edition, about how every L&D team thinks they are underperforming.
The report ends with three clear recommendations for L&D pros:
Understand where you are,
Identify your goals,
Find your own use cases.
Taylor, D. and Vinauskaite, E. (2023). AI in L&D: The State of Play.
👹 Missing links
🤖 Training the android (who may or may not dream)
Here’s a thing that AI is not currently good at: ‘coreference resolution’. I had never heard this term before, but it describes a situation where ‘Sarah, the doctor, stirs her tea’. Each of these three terms in bold refers to the same person, but it’s difficult for a machine to determine this - especially if the terms are paragraphs apart. A team of students at UCL’s Centre for Doctoral Training in Data Intensive Science has been trying to solve this challenge, and the explanation of how they’ve done this is a great insight into how machine learning actually works.
🏠 The debate around working from home rages on
Another big topic this year has been the need to manage hybrid and distributed teams, and navigate the complexities around who, what, where, when and why we should work from an office. In this episode of the podcast Boss Class from The Economist, the CEOs of JPMorgan Chase, Trip.com Group and Slack give their divergent views.
👁️ Maybe one day we’ll look each other in the eye again
There’s an amusing segment in the Boss Class episode above where a representive from Google flies across the world to demo a video conferencing tool. It’s played for LOLZ, but the demo is incredible. Those of us who thought we might spend the rest of our lives on Teams, looking slightly off screen due to the placement of our webcams, can take heart from Google’s Project Starline Prototype - which promises a more intimate connection. Check out the video to see it in action.
👋 And finally…
Long-term readers will know we love a banger here on The L&D Dispatch news desk. So, since we started with a reference to Blade Runner, lets close with the film’s incredible ‘Main Titles’ from Vangelis.
Turn it up loud!
👍 Thanks!
Thanks for reading The L&D Dispatch from Mind Tools! If you’d like to speak to us, work with us, or make a suggestion, you can email custom@mindtools.com.
Or just hit reply to this email!
Hey here’s a thing! You made it to the end! If you love it this much, please do us a solid and hit the ‘Like’ button or forward to a friend.
Ross and I really appreciate it!